Beyond Data to Decision: What you need to know about using Big Data and AI in your business
Beyond Data to Decision: What you need to know about using Big Data and AI in your business
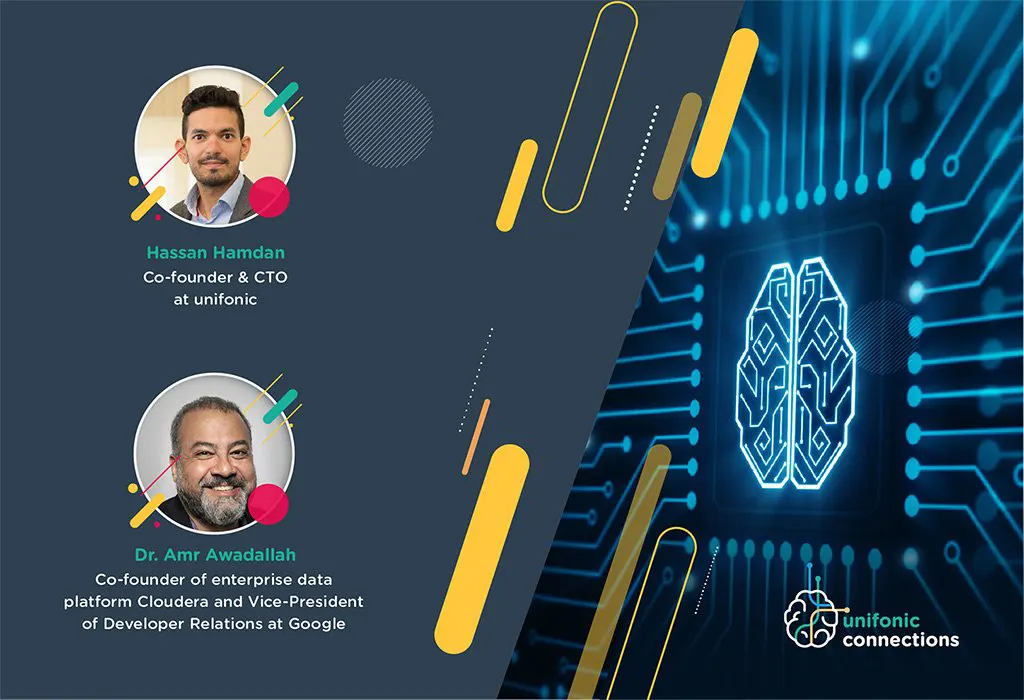
This article is intended to complement unifonic’s Connections webinar, From Data to Decision - How Data and AI Can Drive Innovation in Your Business, in which international expert Dr Amr Awadallah, co-founder of Cloudera and Vice-President at Google, and unifonic co-founder and CTO Hassan Hamdan discuss its potential and its challenges.
Related articles
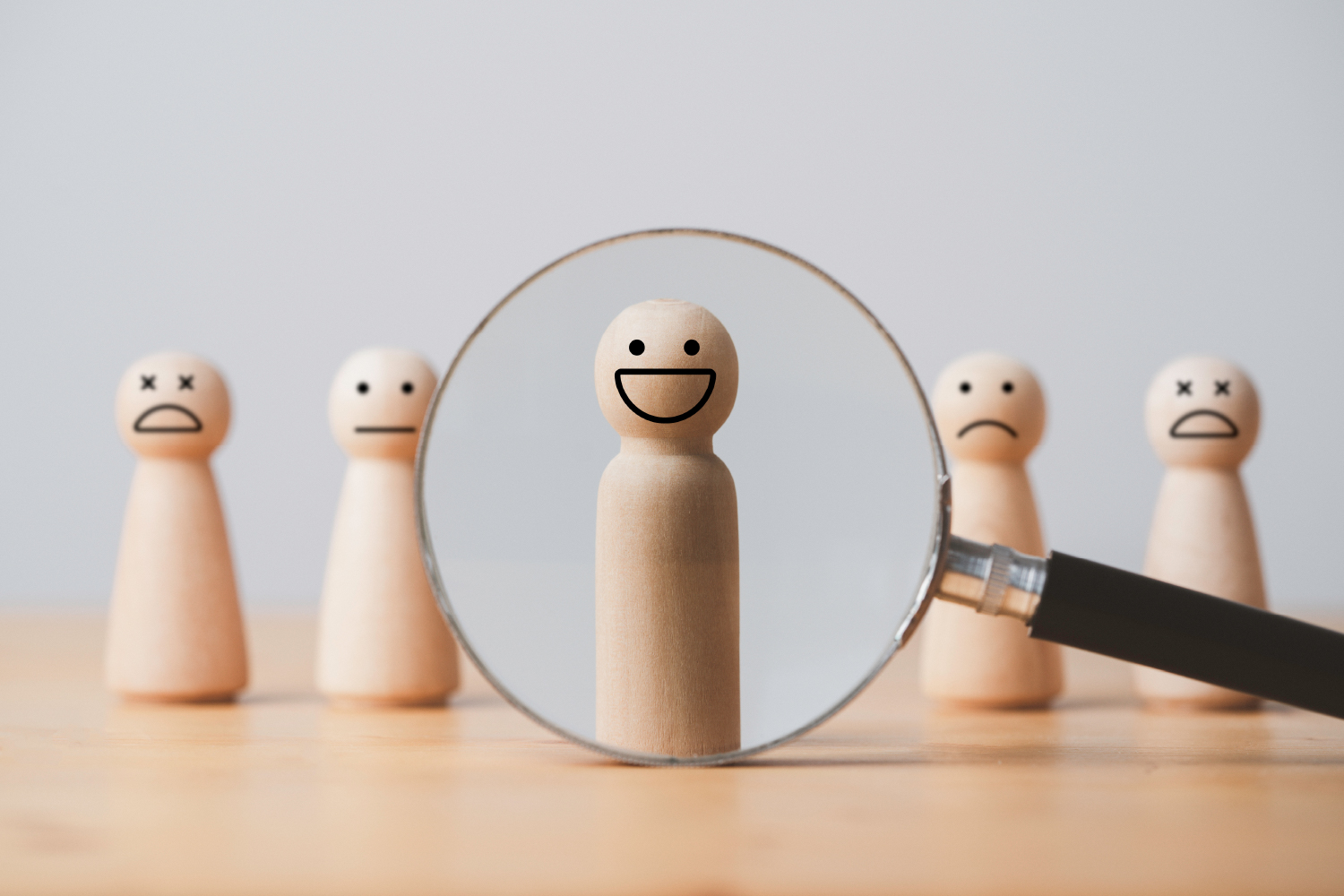
13 January 2023
Unlocking the Power of Personalization & Data Analytics in e-commerce
Personalization and data analytics are powerful tools for e-commerce companies looking to improve communication with their customers. By utilizing customer...
Read more